Deep learning has emerged as one of the most transformative technologies of the 21st century, reshaping industries and pushing the boundaries of what machines can achieve. From healthcare to autonomous vehicles, deep learning is driving innovation at an unprecedented pace. As we look to the future, understanding the trajectory of deep learning becomes crucial for businesses, researchers, and individuals alike.
Deep learning, a subset of machine learning, focuses on teaching computers to process data and learn from it in ways similar to human brains. This technology has already achieved remarkable success in image recognition, natural language processing, and predictive analytics. However, its potential is far from fully realized, and the future holds even more groundbreaking advancements.
This article will explore the future of deep learning, covering its current trends, challenges, and opportunities. We will also discuss how deep learning will impact industries, society, and daily life. Whether you are a tech enthusiast, researcher, or business professional, this article will provide valuable insights into the exciting possibilities ahead.
Read also:Pixel Tracking And Serp Visibility A Comprehensive Guide To Boost Your Online Presence
Table of Contents
- Introduction
- Current Trends in Deep Learning
- Industrial Impact of Deep Learning
- Key Challenges Facing Deep Learning
- Recent Advancements in Deep Learning
- Ethical Considerations in Deep Learning
- Future Applications of Deep Learning
- Data Requirements for Deep Learning
- Collaboration Between Academia and Industry
- Conclusion
Current Trends in Deep Learning
Deep learning continues to evolve rapidly, with several key trends shaping its development. These trends highlight the increasing sophistication and versatility of deep learning models, making them applicable across diverse domains.
1. Edge Computing
Edge computing is becoming increasingly important in deep learning, enabling real-time processing and decision-making. By processing data closer to the source, edge computing reduces latency and bandwidth usage, which is critical for applications like autonomous vehicles and IoT devices.
2. Transfer Learning
Transfer learning allows models trained on one task to be fine-tuned for another, significantly reducing the need for large datasets. This approach is particularly useful in scenarios where data is scarce or expensive to obtain, such as in medical imaging.
Industrial Impact of Deep Learning
Deep learning is revolutionizing industries by automating complex tasks, improving efficiency, and driving innovation. Below are some industries that have already seen significant transformations due to deep learning:
- Healthcare: Deep learning models are being used for disease diagnosis, drug discovery, and personalized medicine.
- Finance: Fraud detection, algorithmic trading, and customer behavior prediction are just a few ways deep learning is transforming the financial sector.
- Retail: Personalized recommendations, inventory management, and customer sentiment analysis are enhancing customer experiences in retail.
Key Challenges Facing Deep Learning
Despite its immense potential, deep learning faces several challenges that need to be addressed for it to reach its full potential.
1. Data Privacy
Deep learning models require vast amounts of data, raising concerns about data privacy and security. Ensuring that data is collected, stored, and processed ethically is a critical challenge.
Read also:Kylie Jenners Dad The Story Behind The Fame
2. Computational Resources
Training deep learning models is computationally intensive, requiring significant resources. Developing more efficient algorithms and hardware is essential to make deep learning more accessible.
Recent Advancements in Deep Learning
In recent years, several advancements have pushed the boundaries of deep learning. These include:
- Transformer Models: Transformers have revolutionized natural language processing, enabling models like GPT-3 and BERT to achieve state-of-the-art performance.
- Reinforcement Learning: This approach combines deep learning with reinforcement learning to enable machines to learn through trial and error, as seen in AlphaGo's success.
Ethical Considerations in Deep Learning
As deep learning becomes more pervasive, ethical considerations become increasingly important. Issues such as bias, transparency, and accountability need to be addressed to ensure that deep learning benefits society as a whole.
1. Bias in Models
Deep learning models can inadvertently perpetuate biases present in their training data. Mitigating these biases is crucial to ensure fairness and equity in AI systems.
2. Transparency
Deep learning models are often criticized for being "black boxes," making it difficult to understand how they arrive at their decisions. Developing more transparent models is essential for building trust in AI systems.
Future Applications of Deep Learning
The future of deep learning is filled with exciting possibilities. Some potential applications include:
- Autonomous Vehicles: Deep learning will play a key role in enabling fully autonomous vehicles, enhancing safety and efficiency on roads.
- Climate Modeling: Deep learning can help improve climate models, providing more accurate predictions and insights into global climate patterns.
Data Requirements for Deep Learning
Data is the lifeblood of deep learning, and ensuring access to high-quality, diverse datasets is crucial for its success. Techniques such as data augmentation and synthetic data generation are being explored to address data limitations.
Collaboration Between Academia and Industry
Collaboration between academia and industry is vital for advancing deep learning. By sharing knowledge and resources, researchers and practitioners can accelerate innovation and overcome challenges more effectively.
Conclusion
The future of deep learning is bright, with immense potential to transform industries and improve lives. By addressing current challenges and leveraging recent advancements, deep learning can continue to drive innovation and create value. As we move forward, it is essential to prioritize ethical considerations and ensure that deep learning benefits society as a whole.
We encourage readers to engage with this topic by leaving comments, sharing insights, and exploring related articles on our site. Together, we can shape the future of deep learning and unlock its full potential.
References:
- Goodfellow, I., Bengio, Y., & Courville, A. (2016). Deep Learning. MIT Press.
- Bengio, Y., Courville, A., & Vincent, P. (2013). Representation Learning: A Review and New Perspectives. IEEE Transactions on Pattern Analysis and Machine Intelligence.

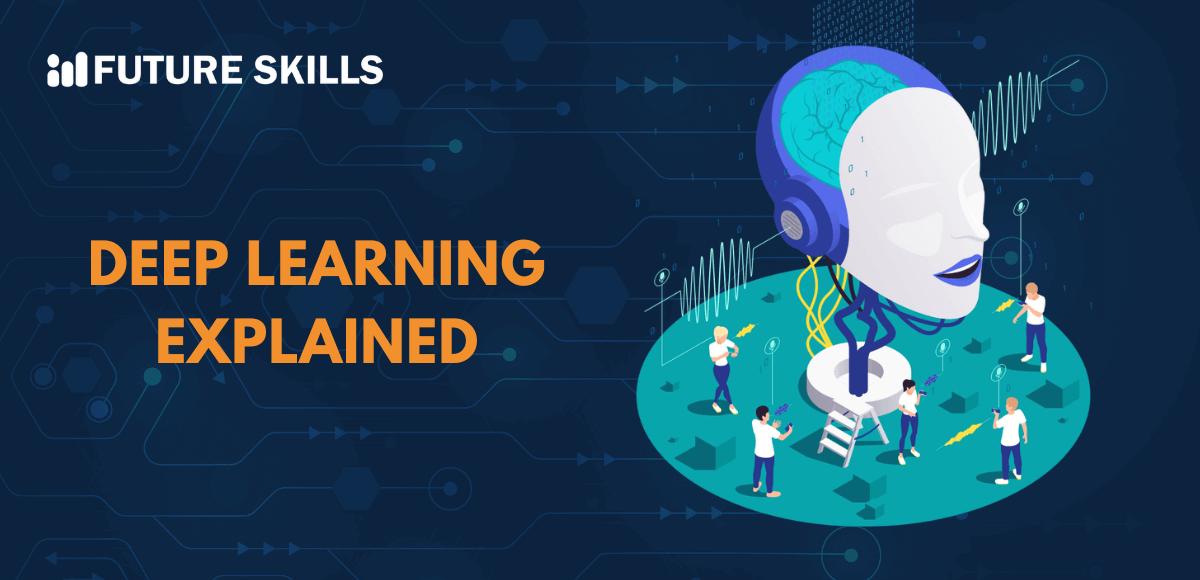
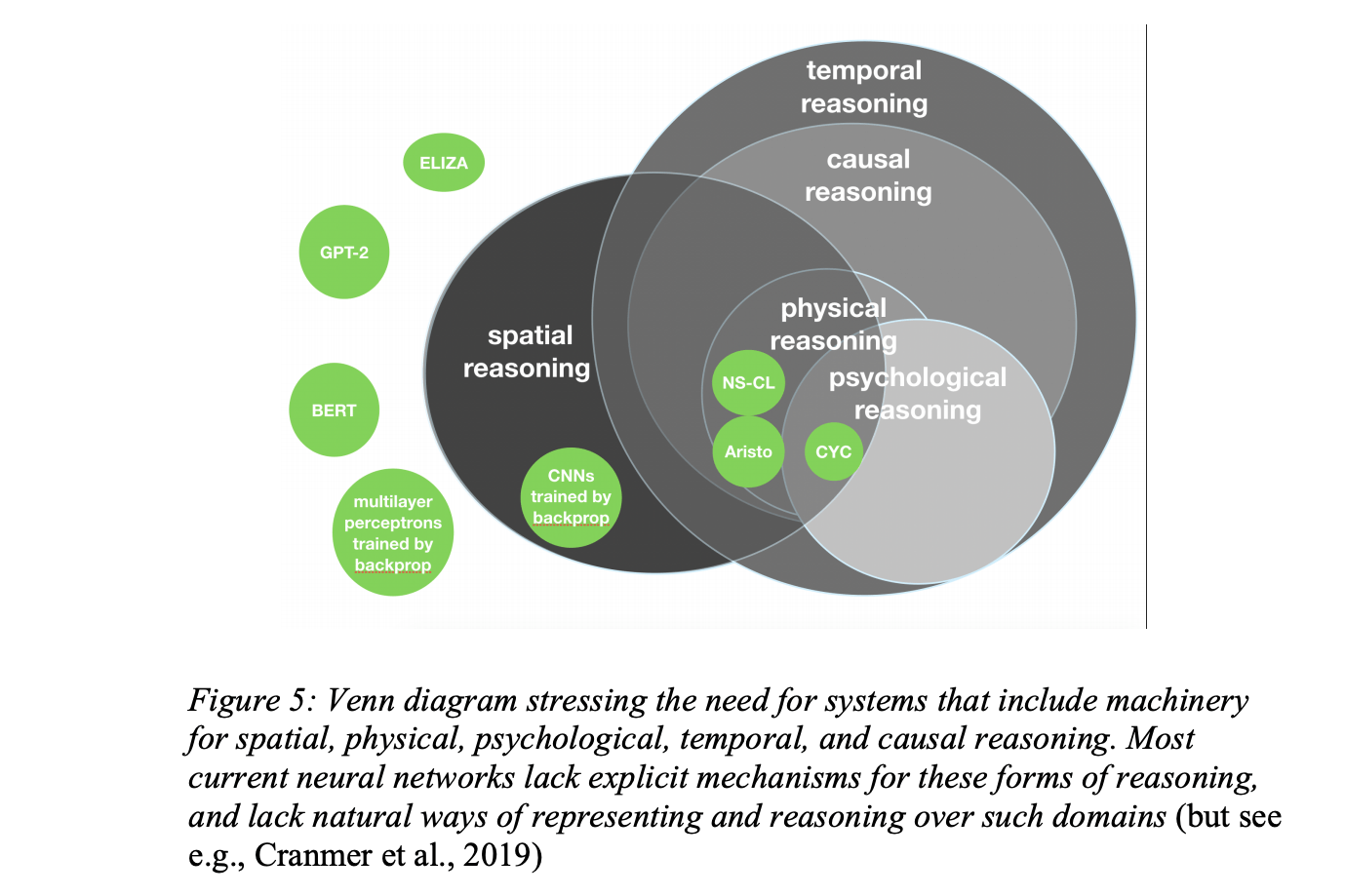